The designs we have reviewed so far have consisted of studying a single behavior across conditions over time. Hayes et al., (1999) refer to these as within-series designs (e.g., B-only, AB, AB*, changing criterion, periodic treatment). But there are other situations in which we may want to compare two different interventions, or the effectiveness of an intervention across different behavior targets, contexts or clients. In this section, we will review two basic designs and their variants: multiple baselines and the alternating treatment designs.
Multiple Baseline
The multiple baseline design is one of the most popular single-case designs, due in part to its simplicity, economy, and relative power to locate and control external sources of error that threaten internal validity. Its flexibility for being able to track and compare a variety of behaviors across multiple contexts also makes it a powerful tool for practitioners.
Several situations lend themselves to using multiple baseline style design for clinical purposes. They include:
- Monitor for extraneous events that may influence your client’s performance, such as maturation or the impact of another training program.
- Determine the impact of your treatment approach on non-targeted behaviors.
- Learn whether the intervention impacts your client’s performance across different communication contexts.
- Evaluate the relative effectiveness of the same intervention across different clients.
In these cases, a multiple baseline approach allows you to ask these questions and may require less data collection effort than individual single-case studies.
How to Construct a Multiple Baseline Design
A multiple baseline design is frequently used to evaluate the impact of an intervention across different target behaviors, as well as, on the same target behavior across different settings or individuals. Most multiple baseline designs consist of a series of separate A|B designs, each devised to track a specific behavior, context or individual. Typically, all the baseline phases start simultaneously, but the phase change from baseline to intervention is delayed or staggered across the different the data series. This staggering of phase changes across the individual A|B data series comprises a central feature of the multiple baseline design. That is, by delaying phase changes across the different data series, noticeable variation of any behavior within a data series can be determined independently, since the phase changes across data series occur at different points in time (Denoia & Tripodi, 2008; Rubin & Babbie, 2015; Morely, 2018).
If the concern is one of internal validity and controlling for extraneous influences, then something like another educational experience, or even normal maturation, should affect the behaviors during the baseline phase. Otherwise, you would expect that the onset of each intervention, occurring at a different point in time, would affect only the specific behavior associated with the intervention. Thus, if there is a change in another data series, then it could be due to an external event coinciding with the phase change or unanticipated, indirect influence of the current intervention on the other behaviors being tracked.
The graph to the right provides a simplified version of a multiple baseline design, displaying three data series (1-3), organized into separate A|B designs with staggered baselines. In data series 1, the phase change starts at the 4th session, in which an immediate response to the intervention can be observed.
To inspect for extraneous influences, look at the baseline data across the individual data series. All baselines appear stable until the phase change in data series 1. In data series 2, there is a consistent increase in the baseline phase behavior at Session 5 (arrow a). Since it is still in the baseline phase, the behavior must be influenced by something external to the data series, perhaps coincidental to the phase change in data series 1, or an indirect result of the onset of the intervention in the preceding data series. However, data series 3 doesn’t seem to change during either of the phase changes in series 1 or 2 (arrows b & c), although the data trends sharply upward after it’s own intervention is applied. One possibility is that the intervention in first data series produced the change in the 2nd behavior target since the behavior change coincided so closely with the onset of the intervention in the first data series. Perhaps the behavior target in the 3rd data series was dissimilar enough to the other behaviors not to have been affected by the intervention as was data series 2.
For a researcher, such a finding would have internal validity implications since it provides an alternative explanation for the expected intervention effect on data series 2 (i.e., intervention on data series 1 was at least partially responsible for changes observed for data series 2). The ability to isolate specific intervention effects across behaviors, settings, and individuals using relatively simple and inexpensive single-case design strategies (e.g., A|B design) makes the multiple baseline design a powerful research tool. For the practitioner, detecting generalization effects across treatment targets might be regarded as a desirable finding since multiple treatment targets could be addressed through a single intervention method.
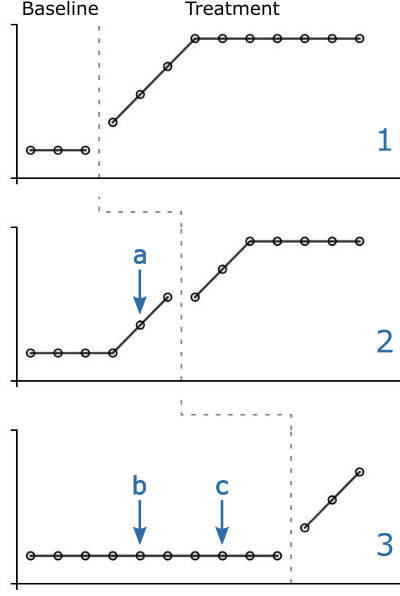
Case Studies: Three Applications of the Multiple Baseline Format
The flexibility of the multiple baseline design comes into play when trying to track and assess clinical cases. The following are three different clinical applications of the multiple baseline design. The first demonstrates how to use it to monitor interventions across three early language targets. The second shows its application to monitoring skill generalization to daily living contexts. The third focuses on assessing progress across multiple articulation targets within and outside the therapy context.
2. Intervention on Agent+Action, Entity+Location & Action+Location Semantic Relations
As you look down across the three data series and assess the individual data paths, what do you find interesting? Data series 3 (action+object) appears to spontaneously improve over time, whereas data series 2 (entity+location) does not. Is the improvement in series 3 due to intervention 1? What evidence do you can you find within the graph to support this hypothesis? What other types of data could you collect to assess for internal validity threats?
From a clinical perspective, do the data here address functional improvements in language production? What kinds of data could you collect to answer Olswang and Bain’s second question about whether the observed changes are important, or not?
It is important to note that this design departs from a true multiple baseline design in a couple of significant ways. First, there is only one treatment, which takes place in a therapy room at SR’s school. The other two graphs report generalization data in which no direct intervention takes place. Graph 2 reports observations made by SR’s primary teacher on his estimates of the frequency of her disfluencies in the classroom. Graph 3 reports SR’s estimate of their stuttering severity over the 48 week observation period. Thus, we are provided with two functional data sources beyond the therapy room.
Second, the lines that extend into the second and third graph panels do not represent treatments occurring in those contexts, but rather, help to structure our interpretations of SR’s functional accomplishments concerning interventions occurring in Graph 1. Both the teacher’s report and the SR’s fluency assessment are considered to be functional probes in that they were obtained outside any treatment setting and collected on a less frequent and somewhat irregular basis.
Treatment and Generalization Evidence for the Effectiveness of SR’s Fluency Program
This clinical application of the multiple baseline design is not intended to address internal validity issues, although the client’s performance under increasing levels of difficulty in the Graph 1 provides some evidence that the intervention is responsible for the observed improvements in SR’s fluency. Besides assessing fluency improvements in the treatment context, what was sought here was evidence that the intervention generalized beyond the therapy context. This design addresses Olswang and Bain’s critical intervention questions about whether an observable change is occurring, and if it is, whether its clinically or functionally important. What proof can you find within the graph to answer these questions? Given the lack of changes in Graphs 2 and 3 over the first 16 weeks of therapy, would you have changed something within your intervention plan to try to obtain faster fluency improvements?
What about Olswang and Bain’s final question about how long should the client receive treatment? Based on these data, should CR stay in therapy, or be released? What’s your evidence? What other kinds of data might you want to collect to help you make treatment decisions about CR?
Treatment and Generalization Evidence for the Correct Production of /v,f,k/
In the first panel, the /v/ phoneme appears to improve quickly in response to its intervention, achieving criteria for word-level performance by session 12, and beginning to approach criteria at the sentence level by session 13. In the second panel, the /ŋ/ phoneme didn’t appear to be affected by the intervention on the /v/ phoneme but was responsive to the intervention specific to that speech sound. In the third panel, the clinician had planned to begin intervention on the /f/ phoneme by session 8 but noticed that it was affected by previous training on the /v/ phoneme. Based on this evidence, she did not intervene and only probed the data. As can be seen, the client /f/ performance improved for the word level at the same rate as /f/ phoneme improvement, providing evidence for a treatment effect carryover from the /v/ to the /f/ phoneme at the word level. However, sentence-level improvements, the /f/ phoneme lagged behind, warranting further intervention. Generalization of /f/ and /v/ for conversation in the classroom context begins to appear in Session 10, but not for /ŋ/.
Determining the Better Intervention: The Alternating Treatment Design
This within-phase design provides an efficient way to assess the relative effectiveness of two or more different treatments on a client’s performance. In the Alternating Treatment Design, each treatment is plotted simultaneously as individual data series on the same graph according to the sessions in which the treatments were applied. The data series are then compared over time regarding their relative effectiveness.
Example of a Alternating Treatment Design
The following are basic considerations for the Alternating Treatment Design:
- An efficient way to compare short-term treatment effects.
- The phase must be long enough (i.e., 3 to 5 observations of each treatment) to observe the effectiveness of one treatment
- Compared to another, but short enough to avoid interaction effects (one treatment affecting the other treatment outcome).
- Consider randomizing the treatment schedule across sessions to avoid order effects (e.g., the treatment outcome for Therapy B is due in part to the fact that Therapy A always preceded Therapy B.
- A common variant of this is the Simultaneous Treatment design in which two treatments are delivered during each session. One needs to be careful that there isn’t significant carryover from one treatment to the other.